In my previous article, I briefly touched upon the concept of “dark data.” Dark data as we know is the untapped digital information that organizations collect and store but generally fail to use for other purposes. One thing we learned is that more than 80% of data is dark, and it provides valuable insights to businesses. Additionally, we are learning how biomimetic artificial Intelligence (AI) and knowledge engineering (KE) play a crucial role in finding and interpreting dark data. Most of us are aware of the capabilities of AI, particularly in automation and data analysis. But do we know that implementing these in specific areas of healthcare can result in significant transformation making it an integral part of healthcare.
Impact of AI on Healthcare Industry
Over the last few decades, the medical field has undergone significant changes with inventions. Such as ultrasound, X-ray imaging, electrocardiograms (ECG), magnetic resonance imaging (MRI), CTs, vaccines and more. However, with the intersection of AI, dark data, and data architecture in the healthcare industry there has been a monumental shift in how healthcare is provided and clinical trials are conducted.
Artificial intelligence in healthcare has become an essential too. Empowering physicians, pathologists, and microbiologists to effectively improve patient outcomes.
One of the most significant impacts of AI in healthcare is its ability to improve diagnostic accuracy. Specifically, AI-powered algorithms can quickly and accurately analyze large amounts of patient data. Like, as medical records, imaging scans, and genetic information.
This remarkable capability enables AI systems to detect patterns and anomalies that human doctors may fail to notice, resulting in faster and more precise diagnoses. As a result, patients can receive timely treatments and expert-level accuracy, leading to better health outcomes.
AI manages patient databases, creates treatment plans, diagnoses illnesses, and even assists with claim processing and financial management. AI can also tackle the lack of medical professionals around the globe by automating administrative tasks, basic patient inquiries, and remote assistance. .
The Integration of AI and Dark Data in Healthcare
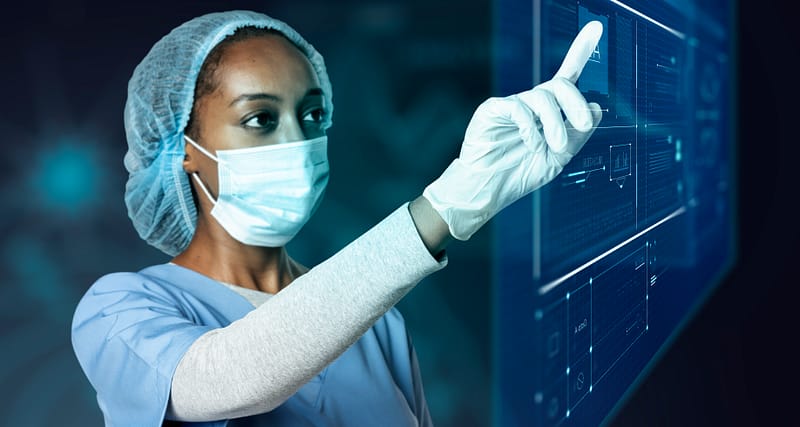
In healthcare, there exists a vast amount of data that is often unused or underutilized. It includes patient records or drug characteristics that are not fully used for other patient management or disease research purposes. The integration of dark data offers a new way for researchers and clinicians to develop better understanding of a disease and revise the treatment process.
The crucial AI algorithms employed for extracting this data include:
Machine Learning (ML): Subset of Artificial Intelligence – its algorithms use data to build models and predict outcomes without programming.
Deep Learning (DL): Subset of ML and mimics the functionality of the human brain. It uses neural networks to extract high-level data and predict outcomes.
Natural Language Processing (NLP): Subfield of AI and ML that focuses on understanding and generating human language.
Clustering Algorithms: Groups similar patient profiles and aids in personalized treatment and detect disease pattern.
Anomaly Detection: Recognizes unusual patterns and aids in early detection of diseases or fraud.
Association Rule Mining: Identifies hidden relationships between medical conditions and patient data guiding targeted treatments.
In comparison to humans, AI algorithms can analyze loads of data from research papers, clinical trials. and treatment outcomes. Its advantage lies in its continuous capabilities improving with each analysis that follows
Let's look at some of the top AI use cases in healthcare that are defining the industry's future.
- Enables Data-Driven Decision-Making – by analyzing unstructured patient records, visual images, and diagnostic data.
- Improves Patient Outcomes – by providing accurate and quick access.
- Early Disease Detection – by analyzing comprehensive patient and family medical history.
- Streamlines Operations – by securely providing patient information online.
- Supports Predictive Analysis – uses data to forecast future outcomes or trends.
- Speed Drug Discovery – is achieved using AI stimulations or computer models to predict the behavior of molecules and their interactions in the pharmaceutical industry.
- Remote Patient Assistance – offers real-time recommendation for life-threatening diseases.
One example of remote patient assistance is offered by ROSEAId , a real-time pathology and research platform that is remotely managed and provides real-time pathology solutions.
This advanced AI-based telepathology platform reduces the turnaround time and creates opportunities to access virtual pathologists in rural areas. Its multimodal LLM approach enhances flow of data required for patient outcomes and trends, leading to efficiency in clinical process.
ROSEAId provides a platform for research to teaching hospitals, revolutionizing new methodologies for cancer detection. It delivers AI-based scoring tools that accurately measure drug effects on trials and get the best therapies to patients.
Currently, the rise in the number of biopsies in the US is expected to increase at a CAGR of 11.12% and cost $20.18 billion by 2030. Also, repeated biopsy procedures and a shortage of pathologists pose challenges to healthcare due to lack of AI implementation.
Another Successful Use Case is in Genomics Research on Multifactorial Disease
TurtleBay Advisory Services has formed a strategic alliance with tech innovator RYAILITI. Which is developing applications in Biopharma for genomics research that have potential to improve drug discovery and precision therapies for any disease.
As featured in Yahoo Finance, a landmark study led by Genzeva performed analysis of samples from patients diagnosed with a multifactorial disorder utilizing a breakthrough process employing whole genome or clinical exome sequencing, a phenotype-driven variant analysis, and the RYAILITI Knowledge Engineering AI Platform (RKE).
The RKE’s multi-dimensional models and ability to reveal hidden “dark” data yielded a remarkable discovery: DNA variants were identified in nearly all patient samples, but not in matched controls in four genes classified as variants of unknown clinical significance (VUS). The findings suggested all four DNA variants apparently play a role in the pathogenesis of the disorder. One DNA variant appears to be a biomarker for the diagnosis of the disease, and two other variants are controlled by the same gene enhancer, indicating a potential hotspot for genomic studies of the pathophysiology of this multifactorial disease.
Research has also shown that actively mitigating risk can lead to noticeable improvements. Therefore, it’s important to keep in mind the benefits of reducing risk in any given situation. Let’s take a look at how risk is managed.
Managing AI Risk
The federal government plays a big role by investing in research and development (R&D) for AI by issuing a joint memorandum for the FY 2024 Budget. The focus is to mitigate AI risk, making AI reliable, fair, and respectful of individuals’ rights and privacy.
In today’s world, risk in AI is the most important factor. Using the VUCA framework for analytics by RYAILITI does not completely eliminate risk, but it can improve problem identification and support an evidence-based approach to managing what we cannot control.
On the contrary, Volatility, Uncertainty, Complexity, and Ambiguity (VUCA) defines the drivers of risk to improve accuracy and is ideal for guiding the architecture of solutions aimed at mitigating and managing AI risk. These approaches are used and deployed to build trustworthy and reliable AI.
To Summarize: The Future of AI in Healthcare
North America, led by the United States, is expected to experience the greatest growth in the digital pathology market. This expansion is being driven by factors, such as increased technology adoption, market demand, and industry challenges that are driving the region to the forefront of digital pathology advancements. In context to the future of AI, the National Academy of Science (NAS) published a report stating that “from the history of neutral networks and artificial intelligence it has come to light that principles of today will be guidelines for the technologies of tomorrow.”
Keep connected to see how TurtleBay Advisory Services can help keep your healthcare business effective.
Kind regards,
Joe
Reference: https://ryailiti.com/ https://www.gartner.com/ https://en.wikipedia.org/wiki/Dark_data https://www.whitehouse.gov/wp-content/uploads/2023/05/National-Artificial-Intelligence-Research-and-Development-Strategic-Plan-2023-Update.pdf https://www.proximie.com/
Visit us @ https://turtlebaybizbooster.com/ https://turtlebayadvisoryservices.com/ https://www.linkedin.com/company/turtlebayadvisoryservices/?lipi=urn%3Ali%3Apage%3Ad_flagship3_messaging_conversation_detail%3B0mx65kQzRgeGJvFA%2BKUHLw%3D%3D https://twitter.com/TurtleBayAdvise https://www.facebook.com/TurtleBayAdvisoryServices/ https://www.instagram.com/turtlebayadvisoryservices/
* If you have any further questions or want more information about how TurtleBay Advisory Services could benefit your organization. Please do not hesitate to book an appointment with us. Contact us at 646-7914511 or sales@turtlebayadvisory.com